Last update: 14 December 2023
Diagnosis
The use of digital technologies for monitoring health-related symptoms started with the development of personal digital devices such as smartphones and smartwatches. These devices can measure vital signs (e.g., heart rate, blood pressure) and movement (e.g., counting steps). These measurements can produce simple health data logs that can provide valuable information for HCPs about patients’ health status.
Treatment
Digital health technologies have the potential to change therapeutic methods and improve clinical outcomes. In this “therapeutic” context they are called “Digital Therapeutics (DTx)”. “Digital therapeutics (DTx) deliver evidence-based therapeutic interventions that are driven by high quality software programmes to prevent, manage, or treat a medical disorder or disease. They are used independently or in concert with medications, devices, or other therapies to optimise patient care and health outcomes.
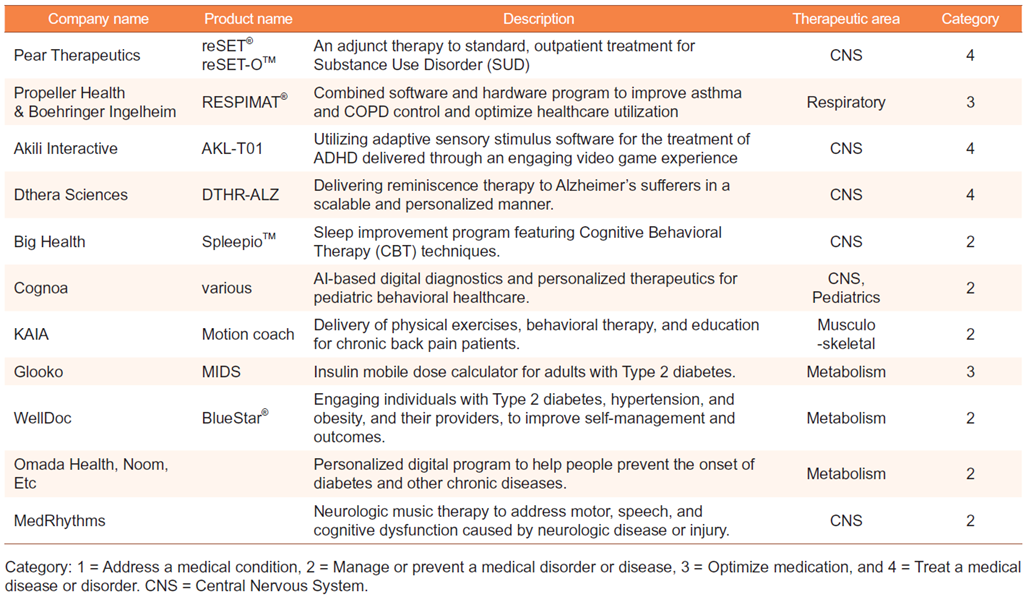
Prevention and Early Diagnosis
Digital health technology can play a role in preventing diseases. The identification of health risks like heart conditions using wearables or mobile applications has been possible due to biomarkers. These early diagnoses can prevent the complications of many chronic conditions.
Prognosis
Another application of digital health is prognosis and prediction of response to a treatment, disease progression, and health condition to help make the right treatment decision. Also, the quality of care can be improved because of improved predictive analytic techniques. These techniques are available using machine learning algorithms and ultimately support development of innovative solutions to improve the quality of care and outcomes [3].
The main challenge in designing a robust infostructure is the compatibility of patient-generated data with existing health data. The popularity of health monitoring technologies and mobile applications has resulted in the creation of health data by patients. Many digital health applications and tools are not designed based on data standards and the generated data cannot be integrated with other health data appropriately and securely.
Learning Resources
Take your skills to new heights by earning certification in emerging fields like Digital Health! Explore the EUPATI Open Classroom and delve into the module Digital Health Applications – Infostructure, Infrastructure to deepen your understanding of the Digital Health landscape. Strengthen your expertise, empowering you to advocate for, engage in discussions, and play a pivotal role in implementing key elements that align with your vision for the healthcare system.
References
[1] Kim J, Campbell AS, Esteban-Fernández De Ávila B, Wang J, Author NB. Wearable biosensors for healthcare monitoring HHS Public Access Author manuscript. Nat Biotechnol. 2019;37(4):389-406. doi:10.1038/s41587-019-0045-y
[2] Chung JY. Digital therapeutics and clinical pharmacology. Translational and Clinical Pharmacology. 2019;27(1):6. doi:10.12793/TCP.2019.27.1.6 [3] Park MPH S, Garcia-Palacios J, Cohen A, Varga Z. DIGITAL RESEARCH: A SUBSTITUTE FOR BIOLOGICAL MODELS? From treatment to prevention: The evolution of digital healthcare AUTHOR